According to The Association of Certified Fraud Executives (ACFE), businesses lose around 5% of their total revenue to fraud every year. In today’s world, fraud is prevalent across all industry sectors. Despite technological advancements and more sophisticated fraud detection systems in place, business fraud is on the rise owing to easy access to information as well as the evolving nature of cybercrime.
Especially during the ongoing pandemic when most people are moving to digital payments, there’s an increased risk of fraudulent activity, and businesses must do everything possible to keep their customers and data safe.
AI and Fraud Detection
Patterns pertaining to fraud evolve continually, making it extremely difficult for institutions to pinpoint and prevent such behavior. If you think you are safe, remember that malicious hackers are attacking computers and networks every 39 seconds.
The emerging attacks are based on machine learning and other automation technologies, making it difficult for legacy software to catch them. One short term solution can be to invest in onboarding tools that assist users to leverage products and help companies to secure 3rd party data.
To keep up with the pace of fraud and prevent it, businesses must shift their focus to AI to build superior fraud prevention models. Here are four ways in which companies can leverage AI for anomaly detection and fraud prevention:
1. Leveraging AI Models for Fraud Prevention
According to the Anti-Fraud Technology Benchmarking Report by the Association of Certified Fraud Examiners (ACFE), organizational spending on AI and machine learning for thwarting online fraud will increase three times by 2021.
To understand how AI can help in fraud detection, it is essential to understand what these technologies refer to. Machine learning is a term used to describe analytic techniques that use datasets to “learn” patterns without any human intervention. AI, on the other hand, refers to a broader application of various types of analytics to achieve different tasks, like demand forecasting, predicting traffic, driving a car, or even detecting fraud. Thus, both the technologies work hand in hand; that is, machine learning is used to build analytics models while AI makes use of these models without any human guidance to achieve the end goal.
For fraud prevention, businesses mainly rely on predictive analytics to detect anomalous behavior and prevent fraud. However, AI makes this process more sophisticated with constant learning that improves the underlying algorithms in time to recognize the evolving patterns of cybercrime.
2. Use Chatbots for Real-Time Dispute Resolution
Chatbots have revolutionized customer service in the past couple of years. AI-enabled chatbots use neural language programming to understand and respond to questions contextually and can handle up to 80 percent of repetitive queries without human assistance.
For instance, The Dufresne Group, a Premier Canadian Home furnishing retailer in Canada, enhanced their customer service with chatbots to sustain bonds with customers amidst the pandemic.
The team is using a conversational chatbot to engage customers and drive sales conversations. The bot captures contact information when the team is out of office. That’s how the sales team has been able to keep sales going 24/7 during the crisis.
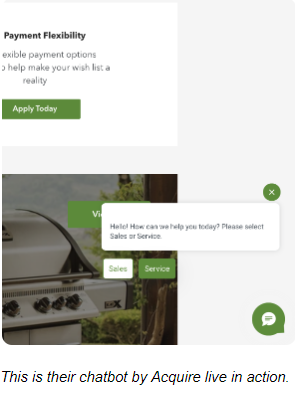
Besides offering 24/7 customer support, smart chatbots also help in fighting fraud. For example, if a customer complains of fraud, a chatbot can instantly block their credit card without any wait time in which more unauthorized transactions may occur.
Bots can also monitor daily transactions and check if there’s any fraudulent or suspicious activity. Powered by machine-learning-based pattern recognition technology, such chatbots can identify unusual behavior or transactions that may be missed by the human eye, and raise a red flag in time to prevent fraudulent transactions.
3. Invest in Predictive Analytics
Most businesses rely on fraud detection models that are based on historical fraud. However, the evolving nature of crime requires firms to continuously evolve their analytics by feeding them with real-time information of the criminal environment. This is especially important in the present times when criminals are using machine learning for curating frauds – as older methods of detection can no longer keep up.
To achieve this objective, institutions worldwide need to come together to build a holistic data repository that takes a broader look at the latest crime patterns. Such knowledge can be developed through intelligence sharing and continuous monitoring of the dark web. The main aim is to shift fraud detection methods from predictive models based on past frauds or rules-based detection to more futuristic analytics based on behavior and well-researched indicators of crime. Such a shift will enable businesses to spot a broad range of suspicious activities and identify new types of frauds to nip them in the bud before they cause severe damage.
4. Use A Combination of Supervised and Unsupervised Machine Learning
Supervised learning is a learning in which we train the machine using well-labeled data or data already tagged with the correct answer. After that, the machine is fed a new set of data for the supervised learning algorithm to analyze and produce the correct outcome from labeled data. Fraud detection based on supervised learning is mainly predictive in nature, but it cannot detect fraud that was not included in the training set. To overcome this challenge, it is essential to integrate supervised and unsupervised learning models for fraud detection.
An unsupervised learning model can detect anomalous behavior, even when there is little or no transaction data. An unsupervised learning model continuously analyzes new data to update itself according to the findings. It does so by noticing patterns to determine whether they’re parts of fraudulent or regular operations.
By combining both processes, it is possible to build a self-learning model that detects the existing types of fraud and predicts new types of crime by outpacing the criminals.
5. Deploy Facial Recognition Systems To Prevent Data Theft
Identity threat is on the rise, which means businesses must adopt advanced solutions to verify user identities and prevent scams. One such method is biometric verification, especially facial recognition, which has proven to be quite useful. However, biometric verification is also prone to attacks like spoofing, deep fakes, 3D mask attacks, makeup attacks, etc. To prevent this, deep-learning-based real-time facial recognition technology has been introduced that matches various parameters, including live features. For banks, such technology can be useful in preventing any suspects from entering the premises.
Digital payment-based businesses can also make use of facial biometric technology to combat payment fraud. They can use online face verification to verify users’ identity in real-time against their picture or ID-card before they proceed to complete the purchase. Face verification can also authenticate the supporting documents provided by users to various financial institutions to prevent fraud or risky transactions.
To Conclude
Fraud negatively impacts businesses and also puts customers at risk. Unfortunately, hardly any industry is insulated from fraud, and it is imperative to adopt futuristic technologies to deter fraudsters or risk unpleasant consequences. AI comes in handy for fraud detection and prevention by automatically detecting suspicious patterns to alert users in time and mitigate fraud.
Author’s Bio:
Dhruv Mehta is a Digital Marketing Professional who works at Acquire and provides solutions in the digital era. In his free time, he loves to write on tech and marketing. He is a frequent contributor to Tweak Your Biz. Connect with him on Twitter or LinkedIn.