Scientists have figured out a way to use an artificial intelligence-based system to make the results of opinion polls more reliable.
Researchers from Spain, at the Universidad Politécnica de Madrid’s Facultad de Informática have established a fuzzy neural network that uses a numerical and categorical imputation method to recreate incomplete datasets. This system attains much better results than the imputation methods currently being used in opinion polling. The results of the research have been published in the specialized Neural Computing & Applications journal.
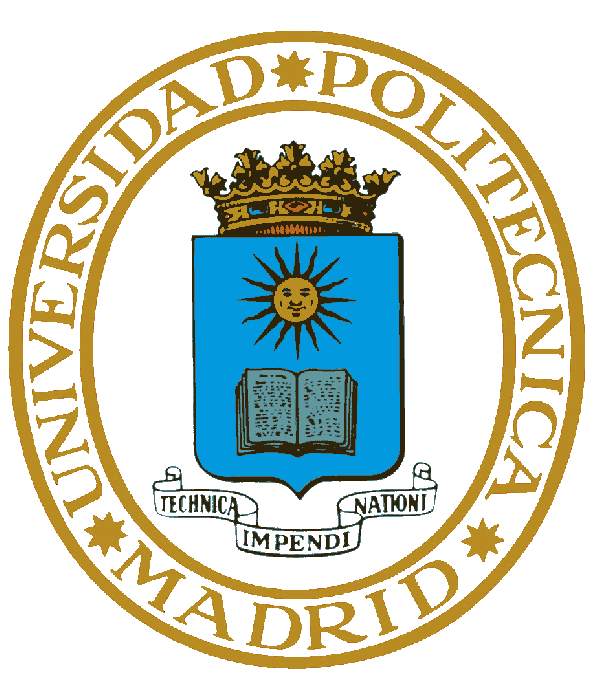
It is well-known that missing data is a problem in most surveys conducted in a host of domains. In political opinion polling inaccuracy can be attributed to how unanswered questions are handled. Imputation is the most frequently used procedure for accounting for missing information.
Imputation methods compute and add the missing data to the sample to get a more thorough dataset. Imputation methods can infer numerical and categorical data.
The researchers Jesús Cardeñosa, of the Artificial Intelligence Department at the Facultad de Informática, and Pilar Rey del Castillo, of the Institute of Fiscal Studies at the Spanish Ministry of Economics, have revised Gabrys and Bargiela’s numerical imputation process to infer categorical data.
Using this system, imputation can be used to determine the voting intention of a person that has not given answers for all of the opinion poll questions. It can supposedly do this with accuracy levels around 90%. This is a huge improvement on existing methods.
Other possible uses for this neural network are medical diagnosis or surveying using categorical variables. Stats geeks are probably salivating at the thought of using a new toy to gather accurate data.
The first stage of this new imputation method is to describe the distances among categories using fuzzy logic. With the support of the neural network that learns from each case, it then decides where each category is located within the different dataset spaces. Finally, it spreads the network architecture to all the data and processes the missing data.
Yes, it’s a system of computerized surveying that learns from different sets of data. It is likely that the reliability of this new system will be scrutinized in the future. That’s not exactly a bad thing at this point.
A system like this needs to be thoroughly vetted before it is put into use in the academic community.