The Defense Advanced Research Projects Agency (DARPA) has kicked off an initiative to develop advanced machine-based visual intelligence capabilities for camera-equipped, unmanned ground vehicles (UGVs).
Current US ground surveillance missions are executed by various military personnel, including Army scouts and Marine Corps Force Recon.
However, the Pentagon hopes to eventually assign such dangerous missions to unmanned systems, thereby removing human troops from harm’s way. Unfortunately, unmanned systems lack a capability that exists only in humans: visual intelligence.
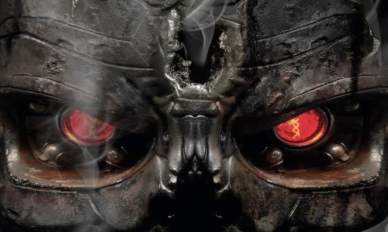
Mind’s Eye – a program aimed at developing a visual intelligence capability for unmanned systems – hopes to overcome this limitation.
“Humans perform a wide range of visual tasks with ease, something no current artificial intelligence can do in a robust way. They have inherently strong spatial judgment and are able to learn new spatiotemporal concepts directly from the visual experience,” the agency explained in an official statement.
“Humans visualize scenes and objects, as well as the actions involving those objects and possess a powerful ability to manipulate those imagined scenes mentally to solve problems. A machine-based implementation of such abilities is broadly applicable to a wide range of applications, including ground surveillance.”
According to DARPA, the joint military community anticipates a “significant increase” in the role of unmanned systems in support of future operations, including persistent stare (or sustained surveillance) missions.
Obviously, a truly transformative capability requires visual intelligence – which would enable autonomous platforms to detect, analyze, report and perhaps sometime in the future, even respond to operationally significant activity.
As such, no less than 12 research teams are now working to code an advanced software subsystem for UGV cameras and sensors.
The teams are also tasked with integrating existing state of the art computer vision and AI, while making “novel contributions” in visual event learning, new spatiotemporal representations, machine-generated envisionment, visual inspection and grounding of visual concepts.