Stanford researchers say they’ve designed the fastest, most accurate algorithm yet for brain-implantable prosthetic systems that let disabled people maneuver computer cursors with their thoughts.
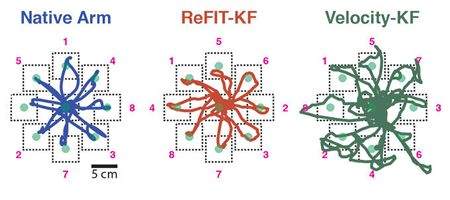
They say the ReFIT algorithm’s speed, accuracy and natural movement aren’t far off those of a real arm, doubling the performance of existing algorithms. And, more than four years after implantation and unlike pther systems, ReFIT is still going strong.
“These findings could lead to greatly improved prosthetic system performance and robustness in paralyzed people, which we are actively pursuing as part of the FDA Phase-I BrainGate2 clinical trial here at Stanford,” says professor Krishna Shenoy.
The system relies on a silicon chip implanted into the brain, which records ‘action potentials’ in neural activity from an array of electrode sensors, and then sends the data to a computer.
The frequency with which action potentials are generated gives the computer key information about the direction and speed of the user’s intended movement.
The ReFIT algorithm that decodes these signals are rather differentfrom earlier models. Usually, brain activity is recorded while the subject moves or imagines moving an arm, and the data is analyzed after the fact.
“Quite a bit of the work in neural prosthetics has focused on this sort of offline reconstruction,” says research associate Dr Vikash Gilja.
The ReFIT system is able to make adjustments on the fly when while guiding the cursor to a target. It can learn from the user’s corrective movements, allowing the cursor to move more precisely than was the case with earlier prosthetics.
To test the new system, the team gave monkeys the task of mentally directing a cursor to a target – an onscreen dot – and holding the cursor there for half a second. ReFIT performed vastly better than previous technology in terms of both speed and accuracy, they say.
The path of the cursor from the starting point to the target was straighter, and it reached the target twice as quickly as earlier systems, achieving 75 to 85 percent of the speed of real arms.
“This paper reports very exciting innovations in closed-loop decoding for brain-machine interfaces,” says Jose Carmena, associate professor of electrical engineering and neuroscience at the University of California, Berkeley.
“These innovations should lead to a significant boost in the control of neuroprosthetic devices and increase the clinical viability of this technology.”