Google researchers have built a neural network comprised of 16,000 computer processors in an effort to simulate the human brain.
The sophisticated neural network was tasked with trawling YouTube, where it managed to teach itself the art of identifying cats as Google engineers fed it random thumbnails of images.
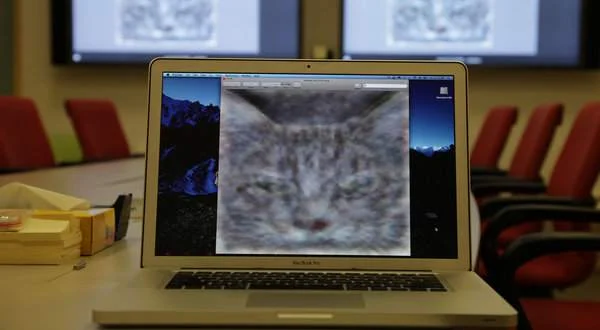
According to theNew York Times, the simulation performed far better than any previous algorithm – approximately doubling its accuracy in recognizing objects in a challenging list of 20,000 distinct items.
Interestingly enough, the software-based neural network also appeared to closely mirror theories developed by biologists which suggest individual neurons are trained inside the brain to detect significant objects.
“The idea is that instead of having teams of researchers trying to find out how to find edges, you instead throw a ton of data at the algorithm and you let the data speak and have the software automatically learn from the data,” explained Dr. Andrew Y. Ng of Stanford University. “We never told it during the training ‘This is a cat.’ It basically invented the concept of a cat. We probably have other ones that are side views of cats.”
As Google fellow Jeff Dean noted, the simulated brain essentially assembled a “dreamlike” digital image of a cat by employing a hierarchy of memory locations to identify general features after analyzing millions of images. Nevertheless, Google scientists believe they have developed a cybernetic cousin of a human brain’s visual cortex.
“The Stanford/Google paper pushes the envelope on the size and scale of neural networks by an order of magnitude over previous efforts,” said David A. Bader, executive director of high-performance computing at the Georgia Tech College of Computing. “The scale of modeling the full human visual cortex may be within reach before the end of the decade.”
For their part, Google researchers remained cautiously optimistic.
“It’d be fantastic if it turns out that all we need to do is take current algorithms and run them bigger, but my gut feeling is that we still don’t quite have the right algorithm yet,” added Dr. Ng.