Are you a hipster, surfer or biker? What is your urban tribe? Your computer may soon be able to tell. Computer scientists at the University of California, San Diego, are developing an algorithm that uses group pictures to determine to which of these groups, or urban tribes, you belong.
So far, the algorithm is 48 percent accurate on average. That’s better than chance–which gets answers right only nine percent of the time–but researchers would like the algorithm perform at least as well as humans would.
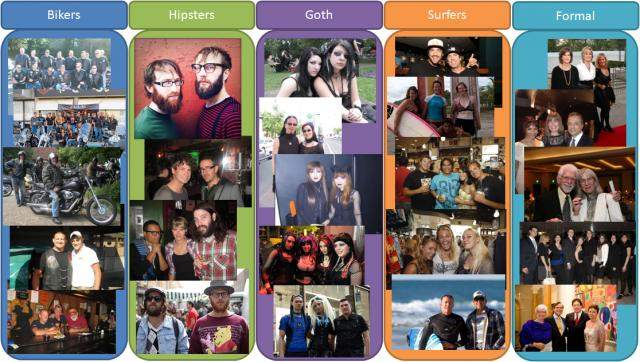
An algorithm able to identify people’s urban tribes would have a wide range of applications, from generating more relevant search results and ads, to allowing social networks to provide better recommendations and content. There also is a growing interest in analyzing footage from cameras installed in public spaces to identify groups rather than individuals.
Computer scientists presented their findings at the British Machine Vision Conference in the United Kingdom this fall.
“This is a first step,” said Serge Belongie, a computer science professor at the Jacobs School of Engineering at the University of California, San Diego, and co-author of the study. “We are scratching the surface to figure out what the signals are.”
This is an extremely difficult problem, Belongie explained, and a 48 percent accuracy rate is actually a very good result. One of the researchers’ insights was to look at group pictures rather than pictures of individuals. They hoped that this would make it easier to pick up social cues, such as clothing and hairdos, to determine people’s tribes based on visuals featuring more than one person.
While humans can recognize urban tribes at a glance, computers cannot. So the algorithm segments each person in six sections—face, head, top of the head (where a hat would be), neck, torso and arms. This method is an example of what’s better known as a “parts and attributes” approach. Computer scientists designed the algorithm to analyze the picture as the sum of its parts and attributes—in this case haircuts, hair color, make up, jewelry and tattoos, for example. The algorithm also analyzes the boxes for color, texture and other factors.
Researchers then let data do the work, feeding the algorithm pictures labeled for the urban tribes they represent—hipsters, surfers, bikers, Goth, etc.—a common machine learning technique. Finally, they fed the algorithm pictures without labels. The computer vision program accurately determined to which urban tribe the pictures belonged 48 percent of the time—better than random. The researcher’s next step is to run the same set of pictures by human users and see how they perform.
In addition, the UC San Diego researchers are working with Lubomir Bourdev, a fomer Ph.D. computer science student at UC Berkeley, and Peter Belhumeur from the Department of Computer Science at Columbia University to improve the analysis of facial features and other attributes within the system.
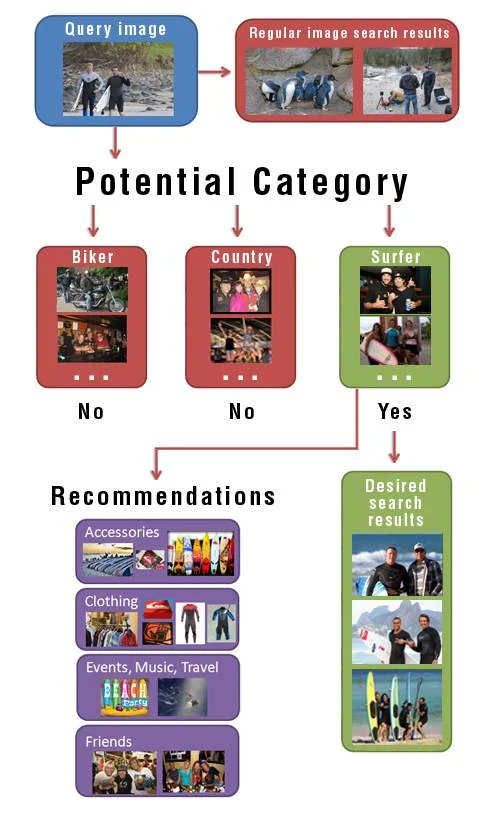
To define urban tribes in the study, computer scientists turned to Wikipedia and selected the eight most popular categories in the encyclopedia’s list of subcultures: biker, country, Goth, heavy metal, hip hop, hipster, raver and surfer. They also included photographs from three common categories for social venues: formal events, dance clubs and casual pubs.
A by-product of their research was the development of an extensive dataset of urban tribe pictures, including hundreds of images, which they plan to make available to other research groups.
In addition to Belongie and Belhumeur, other co-authors on the paper are Ph.D. student Iljung Sam Kwak and Professor David Kriegman, both in the Department of Computer Science and Engineering at UC San Diego, and Ana C. Murillo, from the University of Zaragoza in Spain and currently a visiting scholar at UC San Diego.