Computer scientists say they are now able to reconstruct lost languages using big data computing techniques and machine learning.
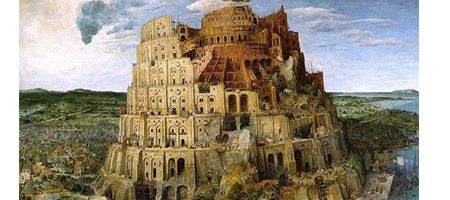
They’ve already used it to reconstruct ancient Proto-Austronesian, which gave rise to languages spoken in Polynesia, among other places. The team now plans to use the same computational model to reconstruct indigenous North American proto-languages.
The model is based on the established linguistic theory that words evolve along the branches of a family tree. Linguists typically use what is known as the ‘comparative method’ to establish relationships between languages, identifying sounds that change with regularity over time to determine whether they share a common mother language.
“What excites me about this system is that it takes so many of the great ideas that linguists have had about historical reconstruction, and it automates them at a new scale: more data, more words, more languages, but less time,” says Dan Klein, an associate professor of computer science at UC Berkeley.
The computational model used probabilistic reasoning – which explores logic and statistics to predict an outcome – to reconstruct more than 600 Proto-Austronesian languages from an existing database of more than 140,000 words.
It replicates what linguists had previously done manually with 85 percent accuracy – and took just hours, rather than years. Using an algorithm known as the Markov chain Monte Carlo sampler, the program sorted through sets of cognates – words in different languages that share a common sound, history and origin – to calculate the odds of which set is derived from which proto-language. At each step, it stored a hypothesized reconstruction for each cognate and each ancestral language.
And the program doesn’t just reveal languages of the past – it can provide clues to how languages might change in future too.
“Our statistical model can be used to answer scientific questions about languages over time, not only to make inferences about the past, but also to extrapolate how language might change in the future,” says Tom Griffiths of UC Berkeley’s Computational Cognitive Science Lab.